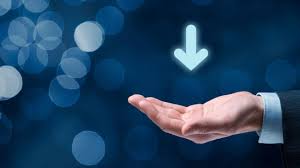
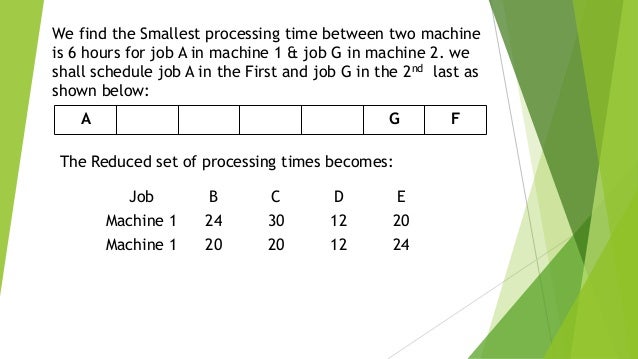
Holistic Decision-Making: This goes hand-in-hand with the bullet point above. This means that if the experiment is carried out in full, we retain all of the statistical power expected based on the experiment setup. No loss of power: When the target duration is reached, the Sequential Testing approach converges with the traditional A/B testing methodology. It’s based entirely on the number of days that have elapsed relative to the planned duration of the experiment. Simplicity: The calculation of Sequential Testing p-values is easy to explain and reproduce, and requires no additional setup from the end user. It’s an adaptation of the Group-Sequential T-Tests for Two Means methodology described here, which meets the following requirements: At Statsig, we selected one that fits in with our A/B testing philosophy. Sequential Testing on Statsigĭifferent approaches exist for computing the adjusted p-values in Sequential Testing. The goal is to enable early decisions without increasing false positive rates by adjusting the significance threshold to effectively raise the bar for what constitutes statistically significant results early on. In Sequential Testing, the p-value computation changes in a way that mitigates the higher risk of false positive rates associated with peeking. In fact, you’ve now increased your chances to 30%. But if you roll it every day for a week, the probability of getting a 1 at least once is much higher than 5%. If you roll it once, you’ll have a 5% (1 in 20) chance of getting a 1. However, ongoing monitoring while waiting for significance leads to a compounding effect of the 5% false positive rate. We do this knowing that there’s a 5% chance that a statistically significant result is actually just random noise. When the p-value is less than 0.05, it’s common practice to reject the null hypothesis and attribute the observed effect to the treatment we’re testing.

In hypothesis testing, we accept a predetermined false positive rate, typically 5% (alpha = 0.05). Limitations of the underlying statistical test Naturally, we want to leverage this powerful capability to make the best decisions as early as possible. These results can then be updated to reflect the most up-to-date insights as data collection continues. Unlike A/B tests conducted in fields like Psychology and Drug Testing, state-of-the-art online experimentation platforms use live data streams and can surface results immediately. This stems from a tension between two aspects of online experimentation: The Need for Sequential TestingĪ common concern when running online A/B tests is the “peeking problem”, the notion that making early ship decisions as soon as statistically significant results are observed leads to inflated false positive rates. Here we outline our approach to Sequential Testing and recommended best practices. This is achieved by adjusting the p-values and confidence intervals to account for the increase in false positive rates associated with continuous monitoring of experiments. We recently released Sequential Testing on Statsig, a much-requested feature that solves the “peeking problem” and shows valid results even when checking on an experiment early.
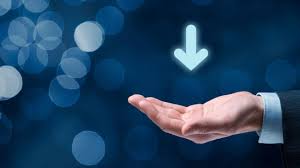